By identifying and localising crops growing in fields, we can inform supply chains about food production months before harvest.
Introduction
The COVID-19 pandemic has highlighted the increasing demand for regular monitoring of agricultural production as a vital instrument to ensure food security and to avoid potential shortages in the food supply chain. Our in-house near-real-time crop identification algorithm helps monitor agricultural activity during the season at a lower operational cost. The exceptional accuracy achieved through the use of satellite data reaches at a level higher than 90% one to two months prior to harvest. Near-real-time identification of crops growing over large areas is also a stepping stone to yield prediction, which would give full visibility on the expected agricultural production at a much earlier stage than it is currently possible and detect anomalies in region-scale agricultural production.
“Hummingbird Technologies is an Artificial Intelligence business that provides advanced crop analytics to its customers by using proprietary Machine Learning algorithms applied to remote sensing captured imagery.
Our expertise allows our customers: to increase their yields, optimise chemical inputs, farm more sustainably and make earlier, more informed decisions.
By pushing the boundaries of science and technology, our mission is to improve the efficiency of global crop production, and to feed the world’s growing population sustainably.”
Will Wells, Hummingbird Technologies’s CEO
Our approach
Remote sensing using satellites has proven to be an invaluable tool for accurate crop mapping across the world. The most conventional way of performing satellite-based crop classifications is based on multi-temporal observations. They have been found to be more effective than a single observation in classifying agricultural fields due to their ability to capture multiple growth stages and varying types of crop structure, allowing for better differentiation between individual crops. Examples of such approaches can be found in the links below [1][2]. However, most of these approaches use the full volume of data for the whole season to make an estimation after the season has ended. Even though such an approach would maximise the accuracy it is not very useful in practice. After the season has ended all the decisions have been made and a crop inventory would have a lot more than archiving value.
Instead, the technique that we developed combines the best of both worlds by (a) using time-series to benefit from crop variation over time (b) being capable to predict crop types as early as mid-season, enabling live forecasting of crop production. This tool, which allows more informed decisions weeks to months before harvest, has been built combining Synthetic Aperture Radar (SAR) data and AI.

Why SAR?
When referring to satellite data, most people envision a typical image provided by GIS applications such as Google Maps (Fig.1). (Optical) images are far from being the only data modality captured from Earth Observation satellites. The list includes infrared images, hyperspectral imagery, LiDAR and SAR.
Figure 1: Canadian Aerial View — Copyright © Google Maps
The farming community is familiar with data that go beyond the commonly used optical images. As a matter of fact, the most common proxy used in agricultural data science is the normalized difference vegetation index (NDVI). NDVI, a synthetic band that combines optical with infrared information, is known to be associated with vegetation health and is widely used to determine management areas for variable rate application of crop inputs [3].
However, both optical and infrared sensors suffer from a common issue in Earth Observation, clouds. Both of them can’t penetrate clouds, hence, any scene that is covered by clouds can not be directly observed during that time. The unpredictability of cloud formation in the atmosphere, as well as the extent (both in space and in time) of cloud cover, imposes severe challenges in their use for this task. A time-series approach would ideally require data acquired in regular intervals or at least a substantial number of cloudless entries. This parameter makes such a tool fully ad-hoc, with an accuracy depending on the geographic location, as well as depending on processes in the atmosphere that can not be controlled and is extremely hard to predict.
In Hummingbird Technologies we have developed a tool with a consistent performance across all geographies and without dependencies from the weather conditions. The solution was using SAR. SAR is a completely different way to generate a picture by actively illuminating the ground using microwaves rather than utilizing the light from the sun as with optical images. Most importantly, SAR penetrates the clouds, it does not depend on weather conditions, it can even capture ‘’images’’ at night (Fig.2)[4]. Therefore, using SAR can make sure that we get data in a regular interval, thus creating a time-series that doesn’t require interpolation or any other tedious and error-prone processing for missing data.
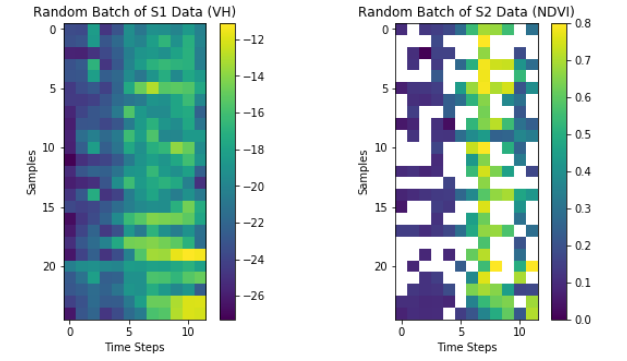
Figure 2 compares the data availability of Sentinel 1 and Sentinel 2. Both Sentinel satellites have been launched from ESA and provide open-source Earth Observation data. The main difference between them is that Sentinel 1 is SAR when Sentinel 2 is optical and infrared. In Figure 2, each column represents a 10-day time period while each row represents one field. The colours quantify two agriculture-relevant data proxies, VH backscatter (in the case of Sentinel 1) and NDVI (in the case of Sentinel 2), while a cell is blank when the data are missing due to clouds. There are two conclusions from this figure. Firstly, Sentinel 2 data is missed over large periods of times, in some cases, months. Secondly, there seems to be a correlation between the proxies estimated from each of the instruments. The conclusion is that using SAR we can get meaningful data, additionally, at regular intervals.
The main reason for getting useful information from SAR is that backscatter is affected most by canopy structure and by other things like vegetation moisture content, wind altering the canopy structure, as well as the properties of the underlying soil [5]. Despite the steep learning curve of SAR, we strongly believe that it constitutes a valuable source of information, which can be much more extensively used in agriculture applications (current SAR applications include ship tracking, disaster detection and ice monitoring), especially ones that would benefit from undisturbed monitoring for large periods of time.
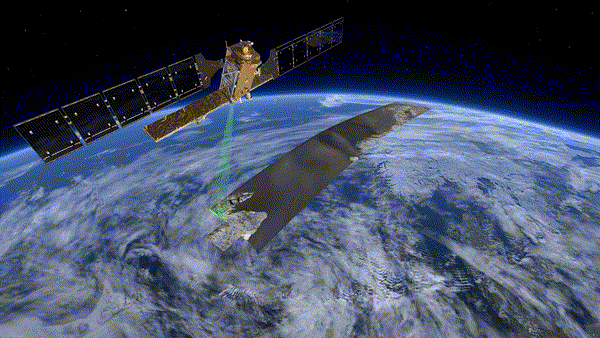
Before we move to a demonstration of this technology at a large scale we should give some practical information about the data used in this analysis. The source of SAR data was Sentinel-1, an ESA instrument which has operated since 2014 and generates open source SAR information in a six-day repeat cycle at the Equator and even more often in higher latitudes. Sentinel-1 GSD is 10 metres per ”pixel”, while pre-processing and denoising of the input was done using Snap open-source toolkit [6]. Figure 3 gives an artistic representation of Sentinel 1 monitoring Earth.
Figure 1: Canadian Aerial View — Copyright © Google Maps
Case Study: Canada
Area of interest and main crops
A pilot study area was selected in the Canadian region of prairies (Fig.4). This is the main agricultural region of Canada, including more than 90% of the total Canadian arable lands, and having a total area of approximately 437 thousand square kilometres. The main crops of Canada are cereals, grasslands, oilseeds, corn (maize) and pulses (e.g. peas, lentils, beans)[7]. The growing season is relatively short, lasting from May to September (Fig.5). This imposes an additional challenge on an automated crop type classification solution by limiting the number of available data points for each field. On the other hand, usually, one crop is grown in each field, hence, there is no need to differentiate between crops growing in the same field.
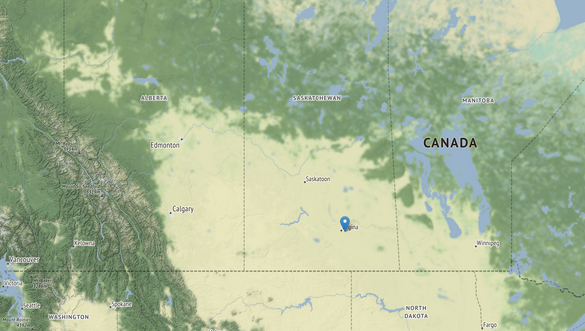
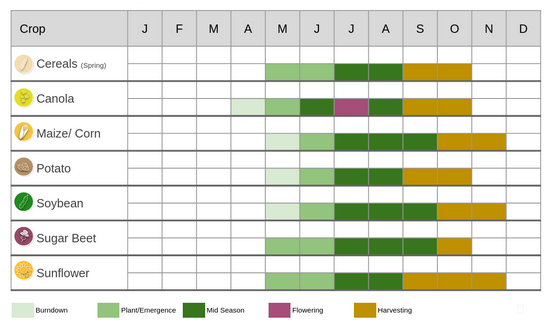
Ground-Truth Labels
Similar to other countries across the globe Canada keeps an inventory covering all major crops on a per-field basis. This archive is updated six months after the end of every season and is released from Agriculture and Agri-Food Canada as a map with a spatial resolution of 30m. More information for Canada’s Annual Crop Inventory can be found in the following links [8][9].
To train, as well as validate and test, our solution, we have downloaded 60,000 field boundaries and crop types for the seasons between 2017 and 2019. The number of fields was selected to generate a dataset with a large number of samples for each one of the main crop types. Moreover, in order to avoid overfitting to the (meteorological, agronomical, etc.) conditions of a specific region in Canada the samples were randomly selected from the whole examined area.
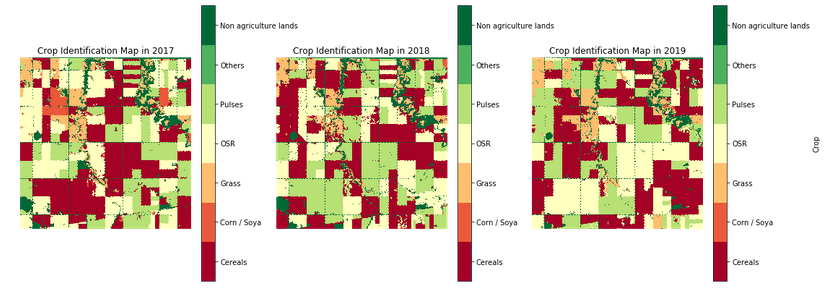
The objective of this study was to confirm that we can automatically generate crop labels for such a large area throughout the growing season (i.e. 7–8 months before the official release) with a high enough accuracy to be useful for downstream commercial applications. Additional goals include identifying the inherent limitations of such a technology, as well as detecting and removing the challenges that hinder its systematic use in a commercial context.

Unique backscatter signatures for each crop
The main hypothesis in this work is that each crop would be associated with a unique (but constant across different seasons) backscatter-vs-time signature, which can be used to differentiate them through a machine learning architecture. This was examined by averaging the SAR backscatter for each crop, and plotting it for 2017, 2018 and 2019 seasons (Figure 7).
It is apparent in Figure 7 that both parts of our hypothesis are valid. Firstly, after a brief period of time in which the backscatter differences are inconclusive, crops, one after another, follow a unique trajectory which renders them identifiable. More specifically, grassland seems to exhibit a quite constant backscattering across the season, something that makes it distinguishable from any other crop type. On the other edge, Canola tends to have the highest increase over the season, which is only reversed a few weeks before harvest. This decrease is also apparent in pulses and cereals while soybean and corn exhibit an S-shape signature with two rather constant zones that are separated over a period of increase. Perhaps most important is the rather small seasonal variation. SAR signatures show consistent and distinct behaviours for every crop type despite varying farm management practices and meteorological conditions in different seasons. These are not random variations of an uncorrelated variable but constitute a unique signature that can be detected.
Figure 7: Evolution of the VH Backscatter (dB) by crop and season
How early?
Achieving near-real-time crop identification does not depend only on the information content of the employed input data but also on when the corresponding time-series start to differentiate from one another. Figure 7 gives some clues on the approximate time that the differences become apparent. By placing our hands in the right edge of Figure 7 and moving to the left it can be concluded that at least one month of observations can be ignored without crops losing their unique signatures. On the other hand, any time earlier than June (i.e. 3 months before harvest) the crop curves appear to be dominated by noise, with only small (and possibly random) differences between them. This time window of 1–3 months before harvest is the range on which SAR can lead to the development of practical applications of near-real-time crop identification.
To show an example of how SAR backscatter changes over time, we have analysed the monthly backscatter of a region (close to the city of Regina, which is in the top-left corner of Figure 8) with large holdings of agricultural land. The temporal profiles of the SAR backscatter from May to August (4 months to 1 month before harvest) are plotted in Figure 8. It is apparent that as the season progresses the fields start differentiating and look more distinct starting from July, i.e. during mid-season (two bottom panels of Figure 8). On the other hand, the differences between 4 and 3 months before harvest are so minute that are hard to distinguish with the naked eye.
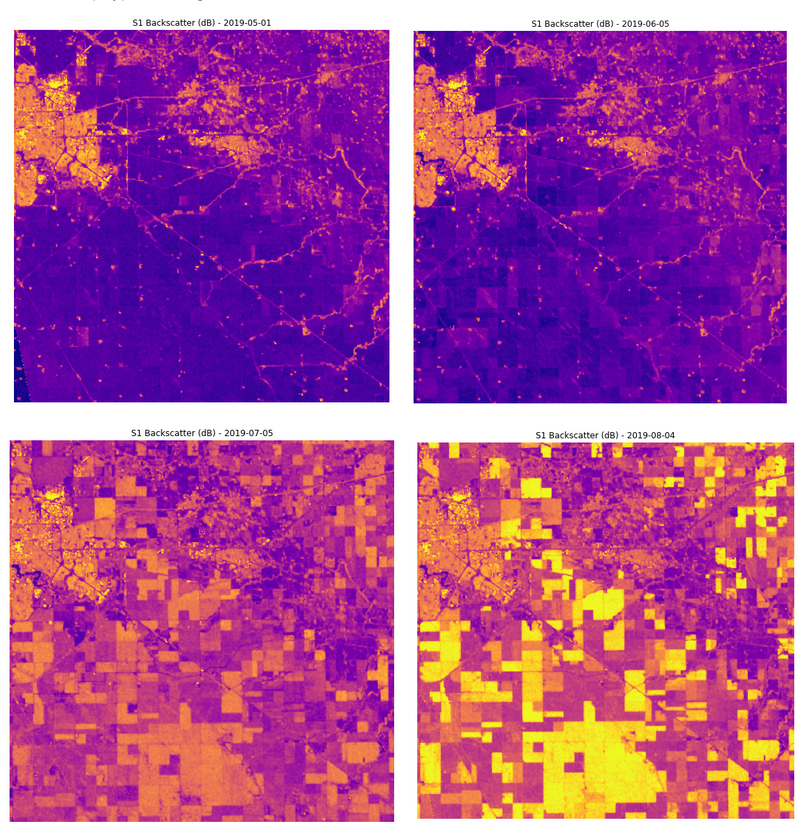
A possible explanation of this SAR property is its correlation to the 3D plant structure. SAR interacts with the full canopy, therefore it is associated with the crop height, the canopy density and the leaf structure. On the other hand, at emergence (when the plants are small) SAR is mainly affected by the soil moisture, which even though it is loosely connected to the crop type it does not hold enough information to make the different crop types distinguishable.
Deep Learning Model
The time-series of SAR data have been the input of a deep learning prototype which was built following the recurrent neural networks (RNN) architecture. RNNs are commonly used to analyse sequential or time-series data and were therefore used as a base layer for the network [10]. A double input model was built combining both Sentinel-1 time-series with optional crop rotation information. Crop rotation information is highly relevant because farmers tend to rotate their crops following a few standard agronomical practices to increase yield potential. Using custom data augmentation and a bespoke architecture we have developed a model that generates predictions at any point during the season. This was tested in weekly intervals for the period between the end of May and end of August. The results are summarised in Figure 9.
The first conclusion of Figure 9 is the confirmation that the last month before harvest can be omitted without any significant loss of accuracy. The performance saturates to 92% around the end of July (6 weeks before harvest), afterwards the improvement is minor, reaching 94% by the end of August. For most practical applications of this technology the accuracy reached by the end of July (or even a few weeks before that) would be enough to generate accurate representations of crop mapping, therefore the improvements over the last month have a little bit more than academic interest. On the other hand, the almost perfectly linear performance gain for the period before indicates the gradual differentiation between crops, which start 3–4 months before harvest and consistently increases over time. One of our main objectives over the next period is to push the boundaries, trying to flatten this part of the curve as much as possible.
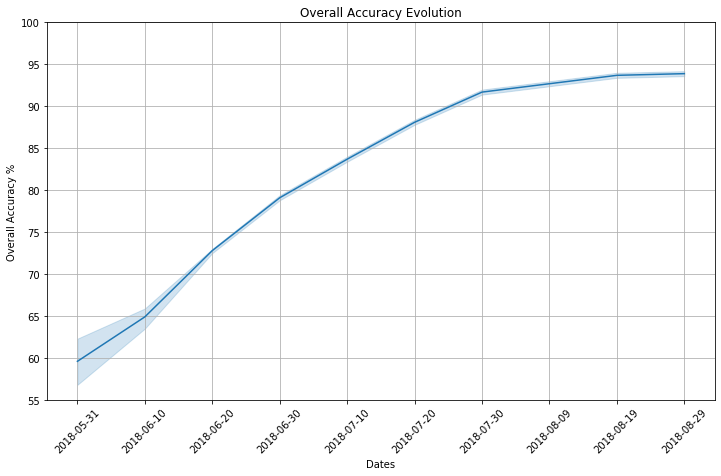
The end result of this technology is crop monitoring maps over large areas. Hummingbird technologies currently provide customers crop type classification maps at a 30 metres resolution, in two setups, (a) one month before harvest and (b) two months before harvest. An example of such a map (in this case, updated every 10 days for demo reasons) is presented in Figure 10. These maps can be delivered both in season across any geography for which ground truth labels are available. We are currently working on a global solution which would use information from multiple geographies to provide an accurate assessment of crops growing in large parts of the world, having as a target to be able to generate a near-real-time worldwide crop map over the near future.
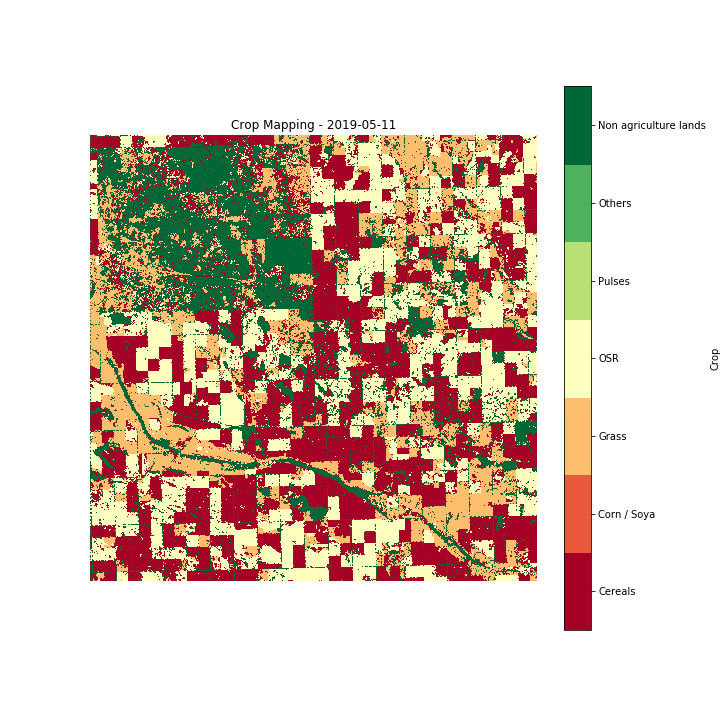
References
[1] Rußwurm, Marc & Körner, Marco. (2017). MULTI-TEMPORAL LAND COVER CLASSIFICATION WITH LONG SHORT-TERM MEMORY NEURAL NETWORKS. ISPRS — International Archives of the Photogrammetry, Remote Sensing and Spatial Information Sciences. XLII-1/W1. 551–558. 10.5194/isprs-archives-XLII-1-W1–551–2017.
[2] Tracy Whelen & Paul Siqueira (2018) Time-series classification of Sentinel-1 agricultural data over North Dakota, Remote Sensing Letters, 9:5, 411–420, DOI: 10.1080/2150704X.2018.1430393
[3] Lee, W., & Searcy, S. (2000, July). Multispectral sensor for detecting nitrogen in corn plants. In ASAE Annual International Meeting, Midwest Express Center, Milwaukee, Wisconsin (pp. 9–12).
[5] Wang, Hongquan & Magagi, Ramata & Goita, Kalifa & Trudel, Melanie & McNairn, Heather & Powers, Jarrett. (2019). Crop Phenology Retrieval via Polarimetric SAR Decomposition and Random Forest Algorithm. Remote Sensing of Environment.
[6] https://step.esa.int/main/toolboxes/snap/
[7]https://www150.statcan.gc.ca/t1/tbl1/en/tv.action?pid=3210000201
[8]T. Fisette et al., “AAFC annual crop inventory,” 2013 Second International Conference on Agro-Geoinformatics (Agro-Geoinformatics), Fairfax, VA, 2013, pp. 270–274, doi: 10.1109/Argo-Geoinformatics.2013.6621920.
[9]https://open.canada.ca/data/en/dataset/ba2645d5-4458-414d-b196-6303ac06c1c9
[10] Ndikumana, E., Ho Tong Minh, D., Baghdadi, N., Courault, D., & Hossard, L. (2018). Deep recurrent neural network for agricultural classification using multitemporal SAR Sentinel-1 for Camargue, France. Remote Sensing, 10(8), 1217.
Article originally posted on medium.com – VIEW HERE